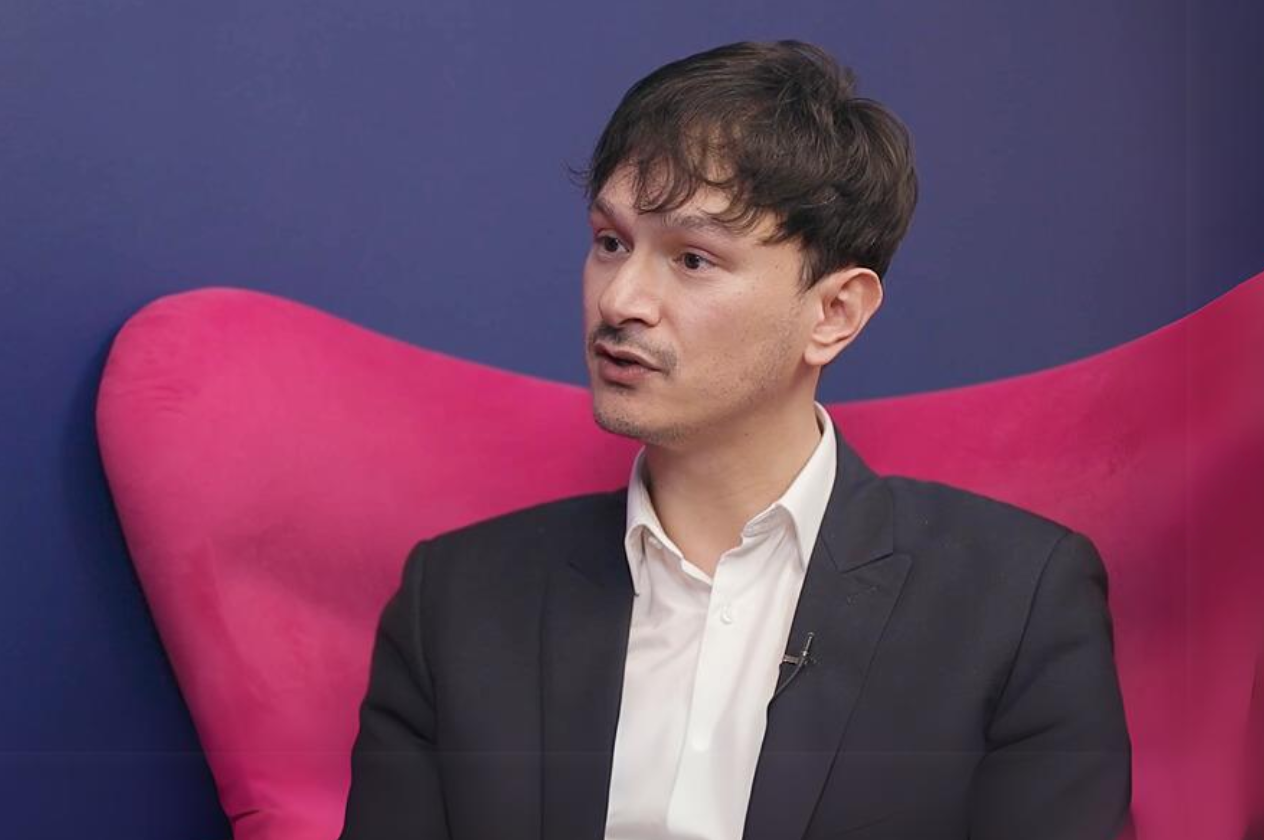
Transcription de l'entretien
Julien Ho-Tong
Partner and Data/AI Strategy & Governance Expert at Artefact
"How to implement a Data Governance strategy."

Julien, can you introduce yourself?
My name is Julien, I’m a partner at Artefact. I work with clients in their business and AI or data transformation. I’ve been working for the past 13 years on energy utilities, manufacturing CPG, luxury industries.
Can you explain what data governance is in a simple way?
We can see data as the gold of your company and your company might produce or use data on a daily basis. It’s just about having this data available with the right level of security, of integrity, of completeness for any kind of users.
Why is data governance essential?
I think it’s important in many ways. We know from benchmarks that the bad data you could have in your company could impact revenue up to 30% which is quite a lot. It could vary in function if you’re working in one industry or another, or with a specific business but it does impact a lot. As an example, if you take bad decisions because you didn’t take the right data and you spent a lot of time to find this data and it wasn’t the right source, you could see that the impact is huge, you spent a lot of time just to take a bad decision. It’s all about having these things sorted out to make sure that you take the right decisions based on the right data.
How and why do companies have bad data?
Very good question. I think there’s not so many companies that say I have to launch an amazing big data transformation program just focused on data governance. So I see that my data is of bad quality, it impacts my efficiency, it impacts my revenue so I have to do something. The starting point would be it already happened. Working on data is something quite new. I think people have been considering data as something we need to focus on for the past 10 years, not more. It starts from a pain point, it hurts the business. So based on that, what could we do. What I see is two different ways to do that. The first is of course to launch a data strategy initiative, so you start defining your data and AI strategy, then you have to work on your organization, then you work on your governance, how you should govern your processes, and on the tech that is behind. That is a way to do that. Another way is a little bit more pragmatic and I think when you want to test and learn to make sure if you need to work on data governance, it’s to start on something way much smaller. You take a usage: for instance, I need a dashboard to check the performance of my products in Germany, so the end product that you will have in between your hands is a dashboard for instance, super neat, super simple. Based on that you will need to work on the processes behind, you will need to make sure that you have the data available, so the different sources of data you need to treat, how you will aggregate that, how you will transform, how you will expose these data in your dashboard. Then you will need people to make that happen, so from the people that would produce this data, people who work on this data, and the people who will expose this data. You have your dashboard at the end of the day, but you see that you need processes, you need data, you need people and you need the tech, so it’s a good way to start a governance initiative – focusing on one very clear usage to see all the impact you will have: people, process, tools, tech, everything. And when you are making sure that it creates value which it is, you can start something at a bit bigger. I think most of the companies I’ve been working with in the past few years, they wanted to start in a small scope, sometimes it was on products, on customers, suppliers, employees, it can be different data domains but starting small, show the value or the impact it has and then you scale it in the company.
How do you manage the risk of teams working independently on a data governance process?
It is mostly what happens and we can’t blame them, because whether you’re working in marketing or in supply chain, you have different needs and at the end of the day you need the work to to be done, so it is very common that you have silos in between the different entities, business lines or data domains. As long as you don’t have a proper data strategy with a proper data governance in place this will happen, so where should I start? And I think the Big Bang thing is not working so well, you will need so much time, so many people to work on that it’s going to cost a lot of money. You need to start somewhere where it hurts and where it can quickly show value. And then it’s also about the culture, because people will see that it works, you will need to convince people that have been working exactly the way that you’ve said in silos, but data is so transversal in the company. Here’s an example, I am in a luxury company, let’s say I create a bag for instance. You will have the innovation or the marketing or the creation part that will think up the bag, they know that these are the trends, they want this kind of bag. Then you have maybe R&D who will work on the raw materials, what we should get, what we should purchase to make that bag. Then you will say “okay this bag, the proof of concept, it’s quite nice, I want to sell it”. Then you need to produce the bag, you need to market the bag, so you’ll need a marketing campaign promotion and stuff. You might also have customer service at the end of the day. You see this is a complete journey, your bag will go across different entities and different teams but the data you’re going to create at each specific point will be used by other people in other entities, other service lines, so how do you make that happen? If I am in R&D and I’m going to create an attribute about a raw material and this will have an impact at the end because they want to have something related to the traceability of the product, I will need this data. If they don’t know that this data they’ll produce will be used at the end of the chain, we’re going to have a problem there. This is why we need to break the silos, we need to think about the product or the customers or the supplier in a full end to end process. And then everyone will have a role to play because the data they will create will be used by someone else.
That’s fascinating! It looks like open data but internal, at the enterprise level. Data that has been produced for economics can be used by people who are working on other subjects, so that’s really great. Whose job is it to break these silos and create common data governance culture and processes?
It’s a bit of everyone’s jobs in the company starting from the top managements. If the top management is not spreading out the culture around data and having good data, you have a problem because if you don’t have their sponsorship in the first place, you won’t have the effort or the bandwidth to give to the team to work on that. So this is the first thing, you need to have a top management that is convinced and that has data governance, data quality or data strategy initiative in their roadmap. Then as you said, it is everybody’s job. If I take again the example of the product, you see that you have different people in different different parts of the company that will have a role. As long as you produce data or you consume data or you make sure that the data is of quality, you will have a role. So again data governance will have an organization pilar, a process pilar and a tech pilar so it is everybody’s role and when you work on your data governance you need to define the role and responsibility all around these processes, the end to end process.
What kind of tools do you need to put a data governance process in place?
This is a question I have a lot. First, I don’t think data governance is directly linked to tools. Thinking that having a top-notch tool will solve data governance is not it. First, you need to work on the processes, the business part and the people. Having said that, of course there are tools to work, to make sure that the rules you have created or the data you are spreading across the company are correct and monitored, you have tools like data cataloging tools or data monitoring tools, you have several things. But this comes at the end of the journey, because if you put bad data in your tool, you know we always say garbage in, garbage out, it’s the same rule all over the process. So whether you’re talking about data cataloging or data quality or master data management, you have a lot of tools, they all do amazing things but it’s all about making sure that you take into account the business part first. Second the existing ecosystem, this not about plugging any kind of tool in your ecosystem, sometimes it will be complicated to integrate that so you need to make sure that this tool is going to answer your business needs, IT needs or data needs. You need to make sure it answers proper needs. The last thing and the most important thing about the tools whether it is a data quality or something else, it’s how you’re going to train the people and show them how it works. You can’t expect to roll out a new tool, whatever it is and people to be super efficient on that, and have a 30% increase of your efficiency. It’s not happening this way. A good example, you’ve all heard about generative AI of course, maybe you heard about a Copilot which is the new tool from Microsoft to help you be more efficient on PowerPoint, Excel and so on, a lot of companies are considering rolling out Copilto at the moment but not all of them are thinking about how I’m going to make sure that the people are going to use it in the proper way or just going to show them how it works. These are new tools you can’t expect anyone to just crack it in a day, so yes the tech is important but first identify for what needs you are going to deploy this tech and how you are going to make sure the people are going to use it the proper way and they are not just alone.
Can you discuss some data governance tools with us?
There are many tools on the market that could fit your your needs. On data cataloging for instance, we have DataGalaxy, we have Collibra, we have OpenMetadata, they are all amazing tools. It just depends on your tech ecosystem, how you want to integrate that, what’s happening and so on. There’s a lot of things also with knowledge sharing, if you want to make sure that the rules you have defined or the list of attributes you have defined can be shared and understood by everyone, of course you can do that with the solutions I have given. Yyou have amazing things that you could do with Jira, with Confluence you have you have great things like this. On master data management, all the tools to manage the referential data or the master data, like Stibo Systems, like Informatica, like TIBCO, Semarchy, they’re also good with that and they have mechanisms to make sure your data is of quality. So for instance making sure that you don’t have duplicates, they have rules that you can set to make sure that if I have a conflict between two customers or two products, it might be this one that might be the right one so you have these kind of things happening. I would start with these ones.
We’ve been talking about data quality a lot, but what about data and AI regulation?
I think we are right in the middle of that. I remember we all got slapped in the face with a GDPR in 2016, it was released in 2016 and it was enforced in 2018 I think as far as I remember, so it was basically on the customer data where we had a lot of standards to respect and to make sure that it was compliant in our systems. In 2023, the AI Act was released and now it’s going to be enforced in one year and a half in 2025. So we have to make sure all our systems are compliant with these rules as it used to be with GDPR, so it is a perfect link to data governance, if data governance is making sure that the data you produce and consume have rules set and that the right people can have access to them or not, and you need to set these rules when you do your data governance. For instance if I take the product data domain, you have a product with a description, a name, you have some things about traceability and if tomorrow in the AI Act it says that you need these controls to be enforced and that you have these rules set in your product database, and it will have an impact on the way people will use them. You’ll have to make sure that they don’t use them in the wrong way, or you could have some some issues at the end of the day so it is very linked to data governance, having these compliance or ethics or whatever rules, set in your data model and data catalog before being used by the users.
It’s real investment and you have to do it because of those regulation but how can you make the most of data governance? If I’m the best in terms of data governance will I be advantaged in some way?
I don’t think there are so many companies that are way ahead in terms of data governance at the moment. Levels of maturity can vary but I’ve never heard about a company where all the data are completely perfect, all the rules are set, super compliant with any regulations so it’s not the case at the moment. However, taking too much time to have these data in line with either your regulations or your own standards, it will have an impact on your time to market, it will have an impact on the way you’re going to sell if your product are less complete for instance or less precise, the client is more likely to get another one from the competitor. It’s also about your brand image. It has so many impacts, not only on the product or the services that you sell, but also on the brand, the company image and so on.
What’s a good reason for spending more time on data governance?
The good reason would be first that not having proper data governance costs a lot of money. It impacts your people. A good example, you’re going to make a dashboard about a product and you want to make sure you have the correct data about this product, for instance a dashboard about the performance of the products. You will spend so much time trying to find the right data to put in your dashboard if it’s not already automatized, so the time you spend finding your right data, the time you spend asking to the right people and maybe there’s not the right people in the company, it’s a lot of time so it’s a lot of money and then eventually you’re going to take a decision based on incorrect data so it has a huge impact. And today a lot of data companies are taking decisions based on uncorrect or very fuzzy data. I don’t know any CEOs I’ve asked the question, who told me I have 100% trust in the decision I made based on the data I have. But it’s normal, we’re still in a phase where we’re realizing these things, I think people have realized that in top management, now it’s about launching this transformation program and these are transformation programs when you work on data governance. It’s a companywide transformation program, it’s going to mobilize a lot of people, it’s going to mobilize business, IT, data people so the whole company. This is why you can’t just launch something so big except if you’ve seen that there is a big interest to do that, you need to start small, show how it works. You need to try, to fail fast if it fails, and maybe start something else or try something else but it works, you just need to make sure how you are going to make that progress with the culture of your company and it is a transformation.
What are the differences between building data governance in a large organization versus a smaller one?
It will vary of course with the kind of business and the kind of industry you’re working on but we have a framework at Artefact that we could apply that pretty much works with all the clients we work with. The first one is when you don’t know that you have bad data and you are immature and so this is the worst case scenario, so it’s not going well but either you don’t care or you don’t know yet. The second are the ones that know the data is bad, they’re conscious about that and they know that they need to progress on that. I would say this is the big majority of the companies today. Then the third level of maturity is you’re conscious about the competence that you have, you are launching initiative about data governance, I would say that this is maybe 20-30% of the companies today. They realized, they know where it hurts and they have launched a few things. And I would say the last level of maturity is when you’re unconscious about all the competence you have, data governance as commodity I would say, everybody’s doing the right things, they know how to find good data, all the processes and tools are perfectly in place, I would say this is like between 1 and 2% of the companies today.
What are some examples of companies that have a near-perfect data governance process?
Of course all the major SaaS companies or the big GAFA of course, they’re known for having good data but they also have some some pain points. Everything is not perfect. When you take Amazon for instance for e-commerce, it is a state-of the-art and a lot of companies doing e-commerce try to get inspiration from this kind of model, but this is a very specific case. I wouldn’t say that when you are a product manufacturer where you have so many data, you are buying things from suppliers, you are adding things on your end and then you’re selling, this is where it gets tricky because there are so many intermediaries in your process or value chain. It gets so complicated to have a good majority of data. Most of them are in the level two, they know they have incorrect data, they know what hurts and they are in progress to launch a transformation program about data governance.
How fast are they moving from step two to step three?
Once again, it depends on the business, the size of the company but data governance initiatives are midterm or long-term initiatives. You can quickly prove the value, I think you should prove the value of data governance working on a small scope or a small data domain for instance during a few months and then I think within three to six months, you can prove the value of data governance. Having the data governance, completely spread it out in the companies on all your data domains, it takes years. But again, you don’t need all these things to be perfectly squared to make sure that your business is running perfectly, you need to focus on where it hurts and where you’re going to get the most value and maybe there are other data domains that could be for a little bit later.
Can you explain how a classic data governance project should work?
Usually when we have the go and usually it is from the top management, so in the C-Suite whether it is the Chief Data Officer, Chief Digital Officer, it could be the CIO, it could be any other person in marketing whatever, but the first thing is to have a C-Suite sponsorship. Once we have done that, you need roles of course in the company. Sometimes these roles are not in place yet because they didn’t start any data governance initiative, maybe data governance is a mix between something that is very IT, working maybe on the data management part or maybe this is something a little bit more business but there’s not this link. The first thing is to make sure do I have the rules internally to work on that and you will need a role that will make business, IT and data work together. It’s not so important if this person is more business or more IY, you need someone that can make that happen. I would say it is more someone that is very collaborative and can move the people. You need someone that you can follow, this is the first thing to have. Then of course you will need to be very sure about who you will mobilize. If you start with a small scope on product for instance, and you will need people from R&D, from marketing, from sales, you need to have stakeholders in all these different service lines, this is the first thing. You will need IT also to make sure that at the end of the day once these processes and these data governance principles are in place, it will live in systems. So how do I make sure that I have the proper person who can talk and that knows what’s happening in the referentials, in your CRM. If I recap, strong sponsorship in the C-Suite, you need someone that knows how to make all these people work together, all these teams work together, and then you will need to have precise stakeholders in all the different entities you will need to to work with.
So what are all the steps in a data governance project?
The first thing is to know what’s happening already, so it would be to do an assessment, what are the usage, what is the culture, who are the people, what are they doing, what are the common pain points. To be pragmatic you need to know where it hurts, where you need to focus. So the first step is making sure where it hurts and what you do at the moment. The second step is to think about the target, so tomorrow if I want to have a proper data governance on my products, if I want to start with products, what are the roles that I’m missing that I don’t have today, what are the roles that I have but they don’t exactly do the proper things, what are the processes and what are the data life cycles under my products, what are the data quality metrics I need to put in place to make sure the data is of quality, what is the architecture in terms of IT, in terms of data and what is the change management I need to foresee to make sure that all that happens. The second step is basically building the target or target operating model around the governance, it is all these steps: process, people, Tech, culture. And the third thing is to make sure that it happens, so you need to roll that out, once again if the usage you have shortlisted or prioritized is having this product performance dashboard, you need to to put that in place so you will of course build a super nice dashboard but you will put all these things in motion, so the processes, the people, you will see things are lacking, things are not working so properly because we miss something and it’s normal, you can’t have like a full 100% thing that is working at day one. You will fail at some point but this is a very iterative process so: one you know where it hurts, two you build a target, three you roll it out with a very practical use case and you learn about what what’s not working, and then you redo on another use case or you redo it on another data domain.
How will generative AI impact data governance in the future?
First I think it’s going to be once the data governance is in place, it is a continuous process, you will you will keep working on that as much as your product or services are going to evolve, the standards, the guidelines, the compliance are going to evolve and the tech is going to evolve. For instance, it will also be transformed with Generative AI. I have a very concrete example at the moment where we are trying to enrich the product data with Gen AI in the product referential which is a a master data management referential, so you have rules that are in this tool but for instance if you have some attributes that have to follow a very strict rule such as “I need this attributes to be a description in 135 characters”, it could take a lot of time you know to to enter that and to respect this kind of lengths in term of words. Using Gen AI is perfect for that, so how do you make sure that you embed Gen AI in your data governance could be a good way to do that or when you have some definitions that are not filled out already about an attributes, you could also use Gen AI for your data catalog to to propose a few things. I think data governance will be more and more a topic on which the companies are going to focus on because they know these are the prerequisites to activate something way much bigger when you want to have like AI factories or data platforms, you need data governance in place. The second thing is generative AI is going to also revolutionalize the data governance part to enrich your data, generate some attributes that are not here yet or when you need to respect a very strict guideline for an attributes like in terms of length, you could use Gen AI, when you want to generate an image for a product that is missing, you could use Gen AI also. So embedding Gen AI in your data governance or master data management is something that is happening already, I’m working on these kind of things at the moment for an electronic product manufacturer and I would say that more and more companies will launch that and they are already doing that. As I said, people like CEOs are conscious about the disquality of data so we can’t allow to lose so much efficiency, so much money on that data, so it’s going to be more and more something to activate, something more complex. If you want to do AI today everybody can do that, but you will most probably have an AI that is not scalable or maybe in silos as you said. If you want to have an AI that is industrialized at scale, you will need data governance in place, otherwise you will continuously try to rework the data that is incorrect.
To conclude, any recommendations to deepen one’s knowledge in Data & AI?
On top of maybe literature, this is about realizing I think do you realize the cost of having bad data in your company and then of course there are many good books about data governance or data management. There’s the DAMA DMBOK book that is pretty well known but you will spend quite a lot of time reading it as it’s pretty big. You need to make sure to align with your company strategy and to be aware of how much efficiency you lose with the bad data and need to make sure what you want to do next.