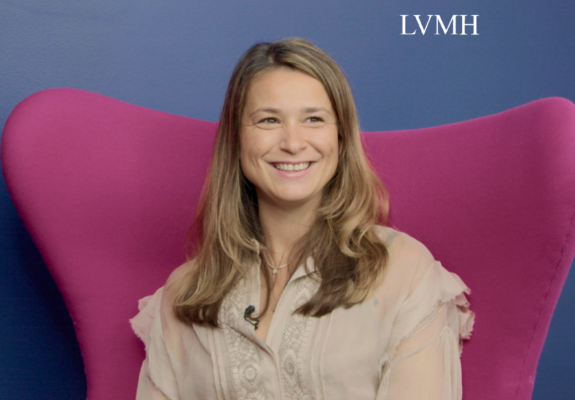
Transcription de l'entretien
Anca MAROLA
Chief Data Officer du Groupe LVMH
"La transformation business passe par l’acculturation à la data."

Anca Marola retrace l’évolution de la maturité data du Groupe LVMH et la façon dont elle a embarqué ses équipes, puis toutes les Maisons du Groupe. Elle donne aussi son point de vue sur la façon dont la création artistique dans le Luxe peut s’enrichir des données.
Anca: I’m Anca Marola, I’m Chief data officer at LVMH. I’ve been working with data for more than 10 years now and with
(00:45) the exciting field of artificial intelligence for I would say about seven years. But back in the day people were calling it mostly statistics and maths, not so much data science, machine learning and so on
Caroline: Why did you get interested about data, data science, coming from this industry?
Anca: You know first of all, my background is in computer science in the beginning in high school but actually I didn’t study computer science afterwards. So I’m not a programmer at all I’m more of a people’s person I would say. I remember
(01:26) back in the day when I had the choice to do my studies in programming or something else I said listen I love programming but what I love more is people and I don’t want to be stuck behind the computer so that’s why I oriented myself towards business school. Therefore, in the beginning I didn’t really think about data so much although I was working with database programming. I was querying uh you know in my first jobs working with data sets but what I was mostly interested about was impact from the customer experience
(01:59) point of view and the business and people’s lives perspective. I think I started my career in marketing research, then I did data mining, digital analytics. And then in the end, ended up going towards artificial intelligence because at the time I think it was fairly obvious to me at one point about seven years ago that it started to massively impact our lives. So if it was starting to be all around us and that’s something that we don’t realize so much it’s actually in all the things that we
(02:33) use every day at our fingertips. You know, be it “do you use Google search? Do you use YouTube? Do you use Spotify, Netflix? All of these things are actually based on AI. So I think for me the trigger was something big is happening, as big as the internet. I think the internet was a huge thing but artificial intelligence was that next thing that was going to transform our lives and I think as a retail industry being in LVMH I knew we had a massive amount of data, because we do own that entire chain of production: you know, we design, we produce
(03:08) We commercialize and we sell to our end customers. Therefore we really have all the value chain and that is why we generate massive amounts of data but we also have that opportunity to apply AI across this entire field so that’s when I first got extremely excited and flagged it to the company that we have to start working on it.
Caroline: Was it easy to introduce this quite mathematical dimension? It’s a bit cool in front of the culture of this company that can be more artistic and more creative.
Anca: for sure it was perceived for a very
(03:54) for a long time it was completely the opposite. When you work in Creative Industries you are very proud to make decisions based on your intuition and that is very important still to have that human feeling that you add to your job I think that’s what really adds the magic but for the most time it was really perceived like incompatible so that was the challenge right what does it mean for us what what can it mean in this type of situation and I think what we tried to form as a vision is data and AI are not here to replace the creativity
(04:32) they’re not here to make decisions instead of the people who are talented they’re extreme Craftsmen they have extreme creativity so data and AI are not here to replace that it’s more about giving them all of the tools and the Automation and the numbers that they need to be able to have that that base to make more important decisions so for us we always start with that when we talk to people in the company for example when we talk to the merchandising teams we say no they’re not it’s not going to do a better job
(05:04) than you but it will help you do your job better because you will save time and you will also have more inspiration at your fingertips more insights and so on so that was for us the journey I think and the messaging and you know I mentioned earlier that I wasn’t necessarily a data scientist so I think that was actually massively worked in my favour in a way in this journey because I think sometimes when you’re an expert you’re very much stuck into the technical specificities in that passion for science but when you work with
(05:36) companies that have an operating way for a very long time it’s not so much about the new technicalities it’s more about why is it important to them and how is their life changing because of this and I think not being that type of geek I was more much more passionate about luxury and fashion and our industry and our products and our creativity so in some way I guess I could speak their own language more than speaking the technical language and I think that was kind of a key to success really understanding your audience the people
(06:09) in front of you and realizing how it can help them in speaking their own language I think sometimes we use a bit too much vocabulary that is hard and difficult to understand so you were the right person to convince them that there are great things to do with artificial intelligence but where did you start we started I would say in the obvious place first of all I think my Approach is always to think big but start small it’s always very hard to convince a company to invest in changing a way it’s operating by pitching a huge
(06:49) project so I think it’s very important to have that test and learn approach it’s important to provide that vision of what it could be even though that Vision sometimes can upset people and can disturb some colleagues but in some way it allows you to already start the change management so first of all forming an opinion that is long-term believing in it and standing by it is really key even if it might be destabilizing but second it’s important to really start small with very concrete pragmatic business oriented use cases
(07:21) what we’ve done was in you know lvmh is a very complex ecosystem we have 75 Brands six divisions so rather than starting to work with everybody on all the topics what we did was to choose two mesons that were particularly excited and interested curious and started to do very concrete business use cases with them so we started in the CRM domain because that’s where we could impact immediately the customer experience so people could also see it they could imagine what it was it wasn’t just in the back end we started by everything
(07:56) that is Predictive Analytics around the customer preferences the future Behavior we’ve put that into the hands of the people in our store so it’s it’s actually quite an interesting Journey because those are the people touching our customers right but they’re also people who are not so much very very aware of artificial intelligence it might be even very scary I think the approach was to give them you know very simple predictive information about their clients so they can better prepared their visits and therefore
(08:28) offer better experiences and that had a big impact both on their own experiences employees they were more efficient but also on the customer experience and of course the business impact we really had a double-digit Improvement on spending retention and so on so that’s how we started then we also started to move into other areas like e-commerce of course e-commerce is a no-brainer because we are so proud of offering amazing experiences in our stores but on the website there’s no one there to greet you right so you have to tailor
(09:02) the experience in a different way data in AI was a no-brainer for that we also moved into a bit the media field with targeting we also started working on product development but more in the enabling of the people developing the products with better tools at their hands for example for casting these are the type of use cases we’ve done and I think that was the the approach to really be able to come and visualize to people what this is and I think that’s one of the major conclusions we’ve had actually this past week had a
(09:36) conversation of where are we in this journey internally and I think the conclusion was aside from all of the projects what matters most is that now people actually can visualize what it is so that’s maybe the advice right to make it visual for people’s day-to-day reality right yeah [Music] so this is the nice story of the use cases that were successful but do you also have example of use cases that were not that were a fail yeah I mean for sure we usually say that you should expect half of your use cases to fail
(10:18) this is by the way for us uh very difficult to accept because we love Perfection we create perfect products so we tend to want everything that we do to always be very successful I think having this mindset that it is important to fail but you need to fail really fast so that you can reach that success faster but it requires a managerial change I would say to be able to empower your teams and let them know that you can also fail for us we’ve had failures in terms of scale as an example maybe the some media use cases didn’t always have
(10:57) enough scale to be industrialized we’ve had also failures in terms of training of people the use case was great the algorithms were working but we didn’t put enough emphasis on training the people using them so the adoption was poor I think there is lots of examples like this but we’ve learned a lot so we tend to take a step back and use those learnings as we move to the next measure and the next use cases and improve really important to hear that that’s okay it’s okay to fail it’s okay if all
(11:33) the use cases are not good ones but talking about the managerial side how do you manage to have the business teams and the data teams work together are they working together in the same rooms are they collaborating that was a key success factor when you do data projects it’s it’s not Tech it’s not business it’s not data it’s really a mix of everything plus HR because it’s change management so actually our first project we from the beginning we put everyone in the same office space we created a
(12:04) little desk space where we had people from HR people from I.T data all sorts of data profiles and the business people and that was the key of being agile because it’s actually very collaborative as a process to create a use case so it’s important to have everyone in the room so that you can make fast decisions and not get stuck we always work in this way that is a cross-functional team even today we’re organized in cross-functional squads I think that as we grow in size because our activity broadens and also the number of use
(12:38) cases the number of mesons grow and our team grows in terms of head count we’ve still tried to split down the team in squads that are still 10 people squads and they are fully integrated with engineering data science business translators plus the business people from Amazon from the group all working together that’s how we are trying to maintain that sort of empowerment and Agility on doing the the daily the daily job how do you manage the priorities for example if the business team have some very important priorities and the
(13:19) technical team the data team don’t doesn’t have the same ones I think this is what we’re solving with cross-functional squads this notion of one roadmap one backlog when you have these people in the room they have to make a compromise and create a common backlog so that was really the solution to having that single instance sometimes it happens that cross projects you also have those types of decision making for one common roadmap so we also put a sort of an extra governance layer that we manage with the executive team to keep
(13:53) track of the progress and make sure that we can arbitrate on the broader priorities another way to to look at it is by prioritizing by value so actually what we’ve done is we’ve created a value office team that has the same methodology to estimate Impact versus is complexity of the business project and that allows us to prioritize a roadmap based on a clear rationale I would say what are your advice for people who would like to reproduce this really interesting story in that team I would say that it’s important to have
(14:35) executive sponsorship even if it’s at small scale it’s important to create and Empower a small agile team that is cross-functional it’s important to be extremely pragmatic and not gimmicky so sometimes there are projects on data AI that are more Innovation oriented I wouldn’t recommend to start with those I would recommend you start with a very important business problem that can be easily visualized by everyone in the company and just focus on that you know rather than doing lots of little projects that might not have a big
(15:08) impact just focus on one do it well takes a lot of effort and then industrialize it so that it becomes day-to-day business and that’s how you get people’s respect and afterwards you can you can multiply that with more teams more use cases more business units but of course the change management aspect is quite crucial so I would really suggest to put a big emphasis on training continuous continuous training not just on the expertise but also on this notion of agility which means empowerment of operationals and that’s
(15:40) not something that all companies are ready to do it’s important to also have that sort of how do you understanding [Music] how do you do two of this training is it like formal training is it more with discussion sharing good practice I would say it’s all of the above first of all I think we transmit passion I always am trying to get people in the team who are truly passionate about what we’re doing because that’s how you impact the people next to you second is every person on the project should always imagine the
(16:21) adoption plan right it’s not just about you’re the expert so you deliver this part for example our data scientists are also supposed to do the explainability part so that they can ensure the adoption by the business people of their algorithms so everyone every single expert has to have an adoption plan and then you can also formalize it and scale it because this is not always so scalable with a data school for example or an academy so this is where we’ve created a data Academy me that provides a big list of trainings both to business
(16:57) people and experts from every business domain on all the topics that is much more scalable and that can reach truly everyone in the Enterprise and we’ve done this with the artifact what’s your next step how do you see your role in three four years I would say that today we’re still in the face where there’s still a big need for business translators I would say in three to four years my dream is that this job no longer exists because the business people are so aware and data driven themselves that they don’t need
(17:37) these people anymore so therefore the the central data team my job becomes much more of an enabler an animation rather than a driver itself so that to me would be the feeling that we have truly succeeded otherwise I would say that what we’re trying to do now is sophisticate even more our approach tailor our approach to luxury and creativity so that means for example not always incentivizing AI on short-term results also incentivizing it on net promoter score how is the customer satisfaction Maybe that’s more important
(18:14) long term it’s proven to be correlated long term to business growth how do we switch a bit that approach in the algorithmic approach to make it more luxurious in itself right aside from that one topic that is emerging a lot is now that we have all the basics covered is everything around ethics we know that also privacy is a big Trend coming up it’s been here for years now but it’s really accelerating a lot globally not just for example in France we have to be much more responsible with the way we
(18:49) collect consent data and what we do with this data and how do we inform the customers about it and how do we control these algorithms because sometimes machine learning is great but sometimes it’s better to opt for something more explainable and controllable rather than a black box so it’s types of thinking that we have currently of how can we give meaning to what we do in a deep away do you feel it’s a strong concern your clients to either talk you about that or is it more about the values you want to
(19:30) bring the regulation also I think it’s both what we say is luxury is privacy it has always been about privacy so the clients give us their trust when they give us their data so it’s important that we treat it very responsibly and we use it for to better their experience we would never do something that doesn’t better their experience that’s a principle but it’s also because I think we are just generally more aware of the impact that data AI has on society and every individual who can play a role in
(20:02) this it’s important that we play it so it’s also a sort of a proactive approach that we want to to establish as professionals that everyone in my opinion should have right how do you impact others and what can you do about it at your own level so you started with the use cases so now how do you scale it when we started initially we were very focused on the business part so we had data scientists we had business translators we didn’t Focus so much on the technique I think now when we want to scale the approach
(20:45) what we’ve done is first of all create and invest much much more in the data platform data governance data collection capabilities because that’s really the the foundation of it all we didn’t do that before a lot now we have teams in place that manage a data platform that is a white label product that is scalable within the missions and also data governance and management policies aside from this we’ve created as well what we call the value office the value office because we’re doing more we have
(21:18) to prioritize it so the value office what they do is they create a sort of assessment that allows us to see what is the Impact versus value and prioritize the roadmap as we want to do more unfortunately we can’t do everything so we have to choose I think that that is really what’s changed between these two stages but as well I think the impact of upskilling at scale has become more crucial if we want to scale your approach you don’t have one year to onboard every single person before the project starts so that’s where a sort of
(21:55) a data Academy has allowed us to pre-prepare the project by upskilling people from the beginning before we arrive on the project so that really allows us to create the sort of understanding and facilitate the projects to make them faster that allows us to to scale today cross-functional teams business people working with better people but other jobs changing are we still talking about business from one side that are from the other side I think that the end goal is to have people both in the regions in the countries in the markets who are
(22:40) data driven themselves plus the business units to be data driven by Nature so that we don’t need the business translators anymore so for me that is the next Frontier localizing the closest to the market as possible and making people more autonomous and that’s how in time the the central data office becomes much more of a factory of reusable assets guidelines trainings inspiration and leveraging those best practices rather than executing up until the end which will be much more in the hands of the business people and the the locales
(23:23) humans very key factors but what about the Technologies how are you sure that you have the right ones the last ones for us it’s always been very important that we are at the Forefront of Technology of innovation because we are at the group level so this is what our Brands expect from us to to bring them the novelty so how do we do that I think it’s multiple ways first it’s through the people that we hire we do have people with PhD in these domains who do active research we do partner with other entities like Polytechnic to co-develop
(24:03) some algorithms we also try to partner with technology companies we do have a strategic partnership with Google on AI that gives us access to people from Google brain for example or deepmind with whom we can exchange on what’s coming next what is the latest breakthrough true that we can leverage in some way but also the respective technological Assets in China that we do exactly the same thing with Alibaba as an example so it’s a mix of having that very strong Partnerships at the group level that are unique that bring value
(24:37) to us and always keeping that eye out and having that Curiosity in the hands of every employee to try and adopt the latest technology and test and learn so I think we do give ourselves that that space for a bit of test and learn so we do have some people always working on some innovation in parallel of an operational project that’s a mindset that we we want to maintain foreign you would like to share with us we have lots of emerging Technologies no not to mention the metaverse recently there will always be emerging Technologies and
(25:21) really sometimes life-changing uh Innovation but it’s important to keep in mind that it’s not so much about the technology it’s about the people and how their lives are changing so any project that we do around it I think the first question that we have to ask ourselves is what does it mean in terms of use cases for them and impact for their lives and and be very focused on this people change management but also the practicality of it for them you know for example the metaverse we are talking about a lot of interesting use cases but
(25:54) in the beginning when it came out it was much more a passion for the technology itself so we see it progressing as well we don’t know where it will head but that’s sort of my my opinion what will you recommend us to read to see is there a talk or book an article that we should read if you want to learn more about data and AI from a business standpoint it’s true that I find the best articles are still the ones done by consulting firms there is this executive guidebook to AI that is done by McKinsey.
(26:36) Quantum black that is quite well done and easy to understand. If you’re interested in the future of how you can control Ai and how you can make it be responsible, a good book would be the ethical algorithm. It’s the latest one that I was reading. otherwise I love following what Yan LeCun is saying so he’s working at Facebook but he’s also pioneered a lot of the emerging breakthroughs in AI as well otherwise the MIT technology review is also a good place to see what’s happening in this field so depends on on what you like but
(27:13) these are my core go-to places.