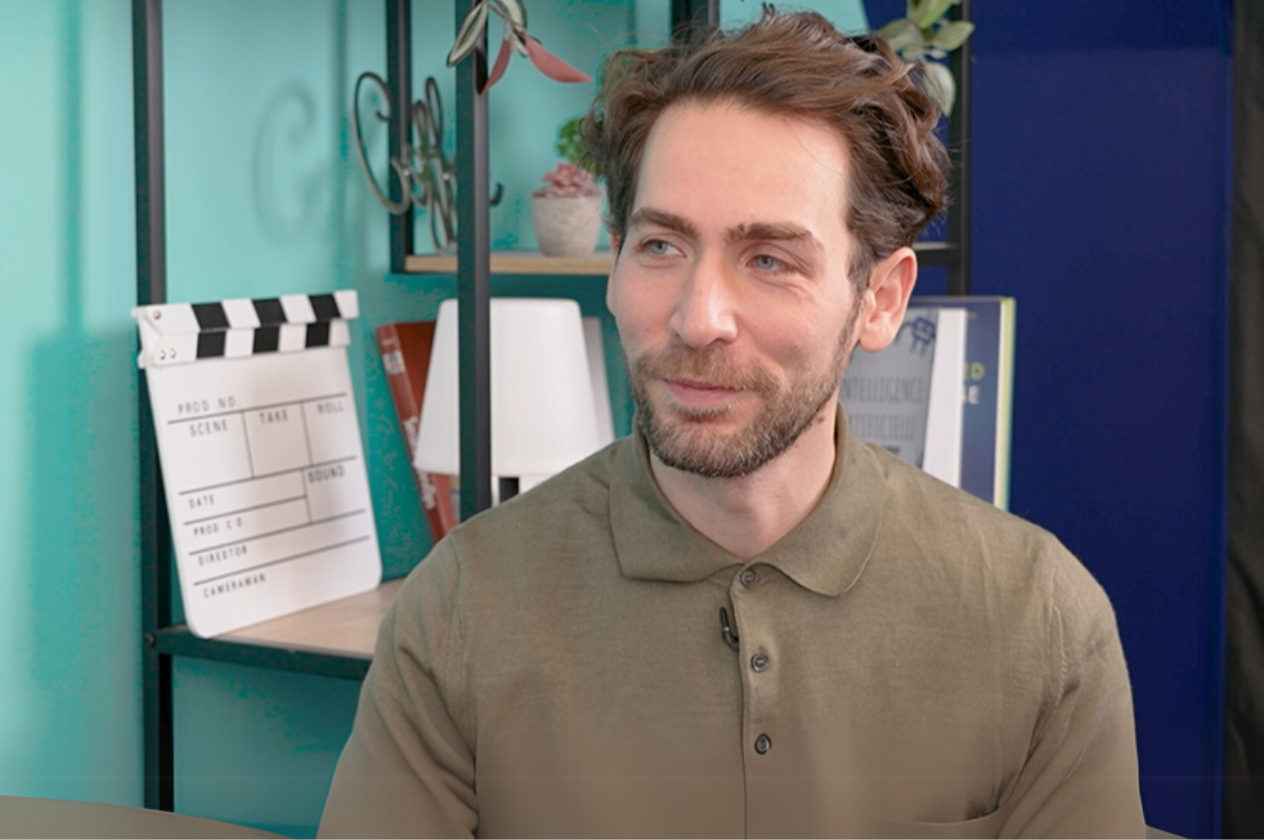
Transcription de l'entretien
Hanan Ouazan
Managing Partner & Global Lead AI Acceleration
"DeepSeek R1: Proving that « bigger is not better »"

Hello everyone, welcome to this new episode of Data Coffee. The concept is simple: we have a guest, Hanan, Managing Partner at Artefact, and a deep topic to discuss today—all over a good coffee. Hello Hanan!
Hello!
How are you?
Very well, and you?
Very well.
You must have been shaken by the arrival of DeepSeek.
Completely shaken!
What Exactly is DeepSeek?
DeepSeek is a model that we’ve been hearing a lot about over the past two years. It’s a model similar to those from OpenAI, Anthropic, Google, and Mistral. It includes different components: a language model, a reasoning model, and more. Recently, it has been making headlines.
Why all the Buzz?
There are many reasons for this. First, we closely follow model developments, especially in Asia. Asian countries have been working extensively on these models, and we’ve noticed a significant acceleration in their releases.
Most recently, around Christmas and New Year, DeepSeek released DeepSeek V3, the third version of their language model. It was already very impressive and competitive with some of the major models on the market. Now, with the R1 model, they have introduced a reasoning model. This is quite rare—a model that not only generates text but also thinks through a sequence of logical steps, a technique known as chain of thought reasoning.
What’s impressive is its efficiency. The announced training cost is around $6 million, which is negligible compared to the hundreds of millions or even a billion dollars spent to train some of the leading models in the US and Europe. Additionally, the cost of inference (running the model) is claimed to be 30 times cheaper than traditional models.
Of course, there are controversies—some argue that not all parameters have been accounted for. But in terms of actual usage, the cost advantage is real. This has sparked strong reactions both in the tech community and financial markets, where we’ve seen stock price movements reflecting this paradigm shift. It challenges the idea that AI development is purely about increasing raw power and suggests that efficiency is just as crucial.
Beyond cost, what’s the real innovation here?
There’s a misconception that DeepSeek is just a copy of OpenAI’s models. While it’s true that models are sometimes trained using larger models as a reference, DeepSeek introduces real innovations—particularly in the training phase.
They have made extensive use of reinforcement learning, a technique where the model learns by trial and error until it reaches an optimal result. Compared to traditional supervised learning, this method is far more energy-efficient, requiring fewer resources and less training time.
This reinforcement learning approach has a dual benefit:
- It makes the model more efficient.
- It naturally incorporates reasoning abilities.
By experimenting to find the right results, the model develops a built-in ability to reflect on its own outputs. This is evident in DeepSeek’s research papers, where they describe the « aha moment »—the point when the model realizes it might have made a mistake. This self-correcting behavior is a direct result of reinforcement learning and sets DeepSeek apart.
Controversies Surrounding DeepSeek
There are also some controversies. Microsoft and OpenAI have confirmed detecting unusual activity on their servers—spikes in requests and queries—which has fueled speculation. Interestingly, when asked certain questions, DeepSeek sometimes responds exactly like OpenAI’s models, further adding to the debate about whether it has been trained on OpenAI data.
That said, DeepSeek is not just an « Asian copy » of American models. There has been significant research behind it, and since it is open source, other AI providers are analyzing its methods to learn from them. This demonstrates that open-source AI is catching up with closed-source models in some areas.
Another major concern is data security. DeepSeek is a Chinese model, meaning that when you use its API directly, the data you send is processed in China. This raises questions about data jurisdiction and security, particularly for companies operating in Europe and the US. Some argue that DeepSeek is still a young startup, and like OpenAI in its early days, its policies might not yet be fully developed. Others worry about long-term data policies.
For those concerned about data security, solutions exist. Companies can use DeepSeek’s open-source version and run it on their own servers in Europe, avoiding data transfer outside their jurisdiction. Cloud providers now offer options to deploy DeepSeek models locally, mitigating the security risks.
Your opinion about Deepseek’s bad buzz?
Another controversy is the built-in bias in DeepSeek’s responses. For example, if you ask DeepSeek about Taiwan, it responds that « Taiwan is a Chinese province. » This is a clear bias reflecting the Chinese government’s stance.
This highlights a broader issue: AI models are not neutral. They are influenced by the perspectives of those who develop them. Similar biases exist elsewhere; for example, in the US, some have started referring to the « Gulf of America, » a term that might not be recognized internationally. This underscores the importance of critical thinking when using AI models.
Ultimately, DeepSeek is not just a simple copy of Western models—it has its own identity and unique innovations.
Thank you very much, Hanan, for this fascinating discussion!
Thank you, and see you soon for another episode of Data Coffee!