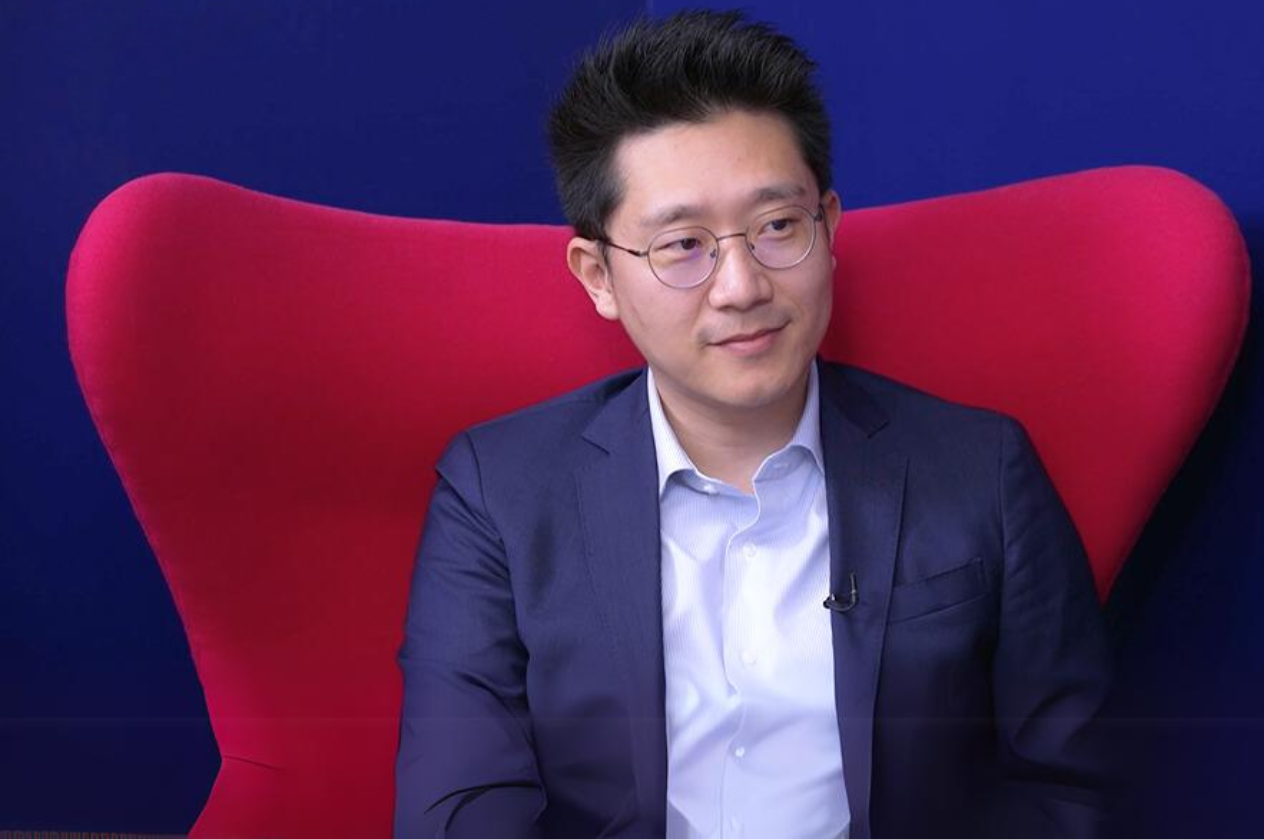
Transcription de l'entretien
Vincent Wang
Chief Digital Officer of Legrand
"Legrand’s digital transformation."

Hello, my name is Caroline Goulard. I’ve created two companies in the field of data visualization. I’ve been working for more than 10 years to build a bridge between humans and data. Today, I’m very happy to host this new episode of The Bridge, the Artefact media that makes data and artificial intelligence understandable for all. We are with Vincent Wang. Hello!
Hello, Caroline.
Can you introduce yourself?
Yeah, sure. I am Vincent Wang, the Chief Digital Officer of Legrand. Legrand is a global specialist in electrical and digital building infrastructure. Me and my team are in charge of the strategy and transformation of the digital side for the entire group.
How did you experience the arrival of generative AI at Legrand? How did it change your approach and your roadmaps?
Oh, that’s a pretty good question because I arrived at Legrand about one year and a couple of months ago, just around the same time generative AI took the world by storm. About a year ago, we published a new digital roadmap for the entire group, focusing on a data-driven customer experience approach, an enhanced channel strategy, and a boosted digital asset approach. A lot of data analytics initiatives were already in the operational parts. When generative AI arrived, it forced us to review our roadmap more regularly. I’d say it impacted three areas.
The first one is about how we do things. For example, before, when we built data catalogs and defined data models, there was a lot of manual work to do. We had to conduct many interviews to understand the ecosystem, the connections, and the data models, and to build processes. But today, with generative AI, we can accelerate the entire process, create common data models, and use AI to do the data mapping. That is a huge gain for us.
The second part is about reviewing business value and effort. For example, we had identified strategic topics for the long term, such as internationalizing our catalog. We produce our catalog in English or French, and translating that into different languages like Spanish, Portuguese, or others would take time, so it was positioned as a mid-term initiative. But with the arrival of generative AI, it became easy to translate catalogs into different languages, so it became a near-term initiative that we launched sooner than planned.
The third part is more about the architecture and how we define the ecosystem with generative AI. I believe we’ve changed how we deal with data. Before, we needed data warehouses and very structured ways to organize data, but today we can adopt a more flexible architecture, connect our data, deal with them, and generate insights from them.
How did you introduce these subjects to top management? How did you explain to them the new goals and challenges?
On that one, I was lucky. It’s a huge opportunity for the entire digital community because, before generative AI, it was quite challenging to explain the benefits of digital initiatives to top management. They are business people, and digital is often considered an enabler rather than a core aspect of the business. But with the arrival of generative AI, this communication and change management became easier because the entire world started talking about it. People heard about generative AI from their families, their children, and in daily interactions.
So, we adopted an approach with top management where we acknowledged that they’d heard about generative AI and had many ideas and questions. We spent a full day together in the same room to align everyone on the vision of generative AI. Everyone had a different level of knowledge, so we made sure they understood the technology. We explored a huge list of potential use cases, showed demos, and case studies. By the end of the day, we had a pretty clear idea of what could be the best use cases to create value for our company, and we agreed on the priorities to launch during the year.
Can you tell us what those use cases are?
Yeah, there are a bunch of very interesting use cases. For example, the first thing that comes to mind is product data. One of the challenges for Legrand is that we have about 200,000 references to manage, sold in about 200 countries. We produce a lot of product data—technical, functional, and marketing descriptions for both B2B and B2C clients—and in about 140 languages. That’s a huge challenge. We’ve done some studies with our teams and found that producing high-quality data for one product takes about four hours per language. Imagine doing that for 40 languages—it’s a massive effort.
One of the most evident use cases we’ve identified is using generative AI to create product data, generate marketing descriptions, and ensure that the generated data remains relevant compared to what was initially created by the R&D team.
You’ve talked about top management, but what about the other members of the organization? How did they adapt to these new technologies? How did your corporate culture help or hinder this?
That’s a very good point. I believe the true power lies in how well the organization understands the new technology. It’s not always about the number of features we put into projects. So, we started by understanding the level of maturity of our people. Some are more tech-savvy than others, and they can grasp the technology faster.
We created three categories for our people:
Those who need to understand generative AI technology.
Those who need to use generative AI applications.
Those who want to create generative AI applications.
We tried to assign people to the appropriate category to better target the delivery of communication and training materials. And, of course, throughout the process, we worked on increasing the maturity of each group.
How did you succeed in doing that?
I’d say our people are very curious about this technology. We started with a couple of cultural awareness sessions. By the way, the CEO of Artefact was invited to one of the sessions to explore what generative AI is and what interesting use cases we’ve seen in the market. We also shared the potential use cases we’d identified within Legrand. People became interested and felt involved in the story. We used storytelling to help people better understand what generative AI is, humanizing the technology so they could think about how it could be applied in their daily lives.
When you say you’re humanizing the technology, do you mean you’re framing it as something that serves humans and helps them? How do you approach that?
First of all, if we look at the features and nature of generative AI, there are many tasks it can perform that resemble human activities. We used a story to introduce this concept. We created a character named Jane—a young person, full of energy, but also mature enough to be reasonable. We said Jane had attended various schools—Harvard, Polytechnique, art school, music school, law school—so she had a lot of knowledge. Jane also speaks almost all the languages of the world—French, English, Spanish, Italian, Chinese, Japanese.
We then asked our people three questions:
Jane has joined Legrand and is willing to work with us. Which team would you like to place her in?
What types of tasks or missions would you assign to Jane so she can really help Legrand?
Who would you like to name as her manager so she can receive the right training and context to do her job well?
That’s so interesting! Were you surprised by the answers?
Yeah, exactly. We created this story to help people get into the right mindset to think about the relevant use cases for generative AI. I believe we’re working on similar things across the organization. One specific thing about Legrand is that we’re in a very distributed organization. We operate in about 200 countries with over 100 different entities. Even though we work in a similar way, there’s still a lot of autonomy and specificities within the local entities.
We’ve been trying to create a central platform that is flexible enough for people to use while also allowing for their local needs. We started with creating awareness for our people to make sure they understood that generative AI is now available and can be used. From there, they could come, discover, and see the concrete applications we had created and imagine how they could be part of the game.
Are you seeing a different level of interest in these generative AI technologies compared to the introduction of previous technologies like email, analytics, or cloud computing? Do people feel differently about generative AI?
Well, let’s say technically, generative AI is born from traditional data and AI algorithms. But in terms of adoption and application, I’d say it’s quite a different technology. It’s a new one because, in terms of accessibility, we’ve never had a technology this democratized. When I shared the use cases with one of our COMEX members, he said it reminded him of VBA, something he used 25 years ago. I told him maybe, but it’s easier now—you don’t need to code or have years of education on digital. You just need to speak, reason, and have a logical way of interacting with generative AI. So, for me, it’s a tremendous opportunity for digital leaders to democratize technology and ensure everyone can benefit from it within the organization.
Would you say it has also changed how teams or organizations are managed? What does it mean to be a manager in the era of generative AI?
That’s a huge topic! We’ve been talking a lot about technology, but for me, the true power of any organization is its connection with people. How can we understand people’s motivations, align goals, work together, and develop the entire team?
In terms of managing teams in the generative AI era, I see two immediate impacts. The first is on how managers spend their energy managing teams. One of the challenges of the modern world is the overwhelming flow of information. Sometimes we get lost in data and information, and we don’t always have the energy to listen to and connect with people. Generative AI helps us process vast amounts of data, which frees up mental space for managers to concentrate on building relationships with their teams.
The second point is that working with generative AI is a bit like managing a team member. You need to provide the right orientation, give proper context, and create the building blocks for the mission. Generative AI has helped many people develop a managerial mindset because it forces them to think like managers.
So, in a way, generative AI has turned many people in our organization into managers. I also believe the role of managers today is to help their teams adopt future technologies like generative AI. When generative AI first arrived, people had mixed reactions—some didn’t believe in it, thinking it was just a buzzword. Others feared it would change everything, and they’d lose their jobs. I don’t believe that’s true. The key is to have a positive mindset and see generative AI as a powerful tool that can augment both individual and collective capabilities.
How do you help your teams develop the skills needed to use generative AI tools?
I’d categorize the skills into two areas. The first is how to better use generative AI tools. The second is about how to build generative AI applications.
For the first one, we’ve launched collaborations between the digital and HR teams to identify the right training materials. We’ve developed a methodology called “Cafe”—Context, Assignment, Format, Experiment—to help people better frame their interactions with generative AI. We tell them that next time they use generative AI, they should think about taking a “cafe.” We’ve also created specific tools with generative AI, like an intelligence system that learns about our products and provides the necessary elements about them.
We’ve trained our client services, sales, and marketing teams to better use this tool with the right prompts and processes. At first, people asked a lot of questions, like how to use the tool or upload files. But over time, they’ve developed expertise. Today, I’m sometimes surprised by their discussions—they even use reference IDs and troubleshoot on their own! That’s amazing progress.
For the second part—developing new applications—we’re taking a learning-by-doing approach. We’ve launched relevant use case projects involving business people, IT teams, architects, and external partners like Artefact. By working on these projects together, our teams are developing the skills to create new applications in the future.
How is your team organized to support these use cases? How do you collaborate with other teams?
That’s a great question. For me, the success of any project or team lies in identifying the business value. So, we’ve adopted a business-driven approach to project management. Our project managers are experienced business people with 20 to 30 years of experience in R&D, marketing, and sales. They not only identify the right use cases but also understand the pain points in our daily operations. These business leaders work closely with different teams to identify priorities, features, and the business value of each project, which helps us build our roadmap.
We start with pilot countries like France, Spain, or Chile, where we have maturity in terms of digital transformation. We get insights and feedback from these teams to create the first version of AI applications. Then we work on rolling out these AI products across different countries and entities.
It sounds like an exciting journey! What are your next challenges? What’s left to do?
We’ve launched some initiatives with our people, but there are three key areas we need to focus on next.
The first one is to continue democratizing generative AI tools. We have a global platform where people can use generative AI capabilities in a safe and secure way, but we need to push more training and help people create use cases that can be put into practice quickly.
The second priority is industrializing the first AI products we’ve created, like the intelligent product agent and the product data quality agent. We want to ensure that not just one or two countries benefit from these tools but that the entire group can use them.
The third priority is focusing on new strategic topics we’ve identified. For example, improving our marketing intelligence by analyzing data from the internet and studies to guide our business and product strategies. We also want to improve pricing management to maintain margins while increasing volume and enhancing the customer experience using client data. These are just a few examples, but we’ve got a long list of exciting projects in the pipeline.
When you look back over the past year and a half, what was the hardest part for you?
Maybe I should start with the easiest part, which was the passion and interest from our people at almost every level, starting with top management. Surprisingly, they were the first users of our internal generative AI tools, which is unusual because they are usually the last to adopt digital tools. The management teams across different countries and entities were also very interested. Not only did they try to understand how to use the technology to create immediate business impact, but they also helped their teams grow their understanding and maturity in AI.
For the hardest part, I’d say it was aligning the vision and business priorities. In a large organization, it can be tempting to let everyone pursue their own initiatives, but that’s not the most efficient way. The challenge is creating a group-wide framework and platform that different entities can use while allowing them to create their own solutions.
Can you tell us more about your first use cases? I’m thinking about the two use cases you identified with top management.
The first one is the product data quality agent, which we’ve named « Gaia. » The second one is the intelligent product agent, which we’ve named « Elia. »
Gaia is a tool that fetches basic information from our product database and develops comprehensive marketing descriptions for our products, whether for B2B or B2C audiences. These descriptions vary in length so we can use them in different scenarios—whether it’s for e-commerce, mobile platforms, distributors, or technical guides for installers. Creating good-quality data manually would have taken a lot of effort, but with Gaia, it’s almost instantaneous. We still have processes in place to review and validate the content, but thanks to G, we’ve reduced the time spent managing product data for a single reference in one language from four hours to just half an hour, which is a significant improvement for the entire team.
We’re currently in the UAT (User Acceptance Testing) phase and collecting feedback to integrate into the next version of the tool.
As for Elia, it’s a generative AI agent that has learned Legrand’s product catalog. For example, if a client has just bought a new electric vehicle and is looking for a compatible EV charging station, they can ask Elia for recommendations. Elia can analyze different models, compatibility with the client’s home, and compliance with city regulations. Or, if a sales manager has a new catalog to share with clients, Elia can provide summaries of the latest products and help the manager master the key points that need to be pushed forward.
What was the process for these use cases? What were the steps?
We spent a whole day with COMEX to prepare for this. We identified some quick wins and built demos, including the product agent and data generation tool. COMEX members were very interested and asked us to create the projects. So, we launched a collaboration with Artefact and different business teams, especially in France, to create prototypes and make them into MVPs (Minimum Viable Products). We started using them with early adopters, mainly in Europe and France. We received great feedback, and users loved the new AI products.
Now, we’re working on industrializing these two products—creating a stable platform with the right infrastructure, cybersecurity, and legal compliance. We’re pushing this roadmap to the entire group.
Do you think these changes from generative AI will last, or do you think it’s just a fashionable buzzword that will disappear soon?
A year ago, we might have thought it was just a buzzword. But today, with the tools we’ve created and the daily usage we’ve seen, it’s clear that generative AI is here to stay. It creates tremendous opportunities but also challenges. In terms of both data quantity and quality, generative AI will change how we manage data.
At first, I used generative AI mainly to help with writing emails or drafting messages. But over time, it led to deeper discussions, and we started realizing that generative AI could change our workflows significantly. Today, we’re sure it’s a lasting technology with new models, capabilities, and powerful features. It’s not the answer to every question, but it can be a powerful answer to relevant questions. It has also changed the relationship between people and technology—everyone can now act as a manager with the help of these tools.
If you had to conclude our conversation today, what would you say?
I’d share a philosophy for myself and my peers. Take generative AI as a tool and an opportunity to re-inject fun into work. Over the past few months, with the use of generative AI, I’ve been able to reduce my mental workload and drive things in more dimensions. Sometimes, I feel like I have more space and energy to really think and reflect. So, I see this as an opportunity to create more freedom and playful engagement with technology for myself, my team, and the organization. That’s a memo to myself too.
Thank you very much, Vincent!
Thank you, Caroline. I hope you enjoyed this episode. See you soon for a new episode of The Bridge.